搭建运行脑仿真网络#
作者: Shawn
本文主要介绍如何搭建并运行一个脑仿真网络。
定义网络#
首先使用 neurai.config.set_platform
来设置平台,可选的平台有 cpu, gpu, apu ,如果选择 apu, 具体可参考用户手册中 APU平台运行示例
。
然后通过创建 neurai.nn.snet.SNet
的子类来定义一个网络:
from neurai.config import set_platform
set_platform(platform='gpu')
from neurai import nn
from neurai.const import ConnectRepr
class SimNet(nn.SNet):
def setup(self):
self.lif0 = nn.ExpLIF(size=100, v_init=-55, V_rest=-60., V_th=-50., V_reset=-60., tau=20., I_e=0.)
self.lif1 = nn.ExpLIF(size=100, v_init=-55, V_rest=-60., V_th=-50., V_reset=-60., tau=20., I_e=0.)
self.poisson = nn.PoissonGenerator(size=100, rate=1000)
self.dc = nn.DCGenerator(size=100, amplitude=50, start=0.5, stop=1.5)
self.synapse0 = nn.StaticSynapse(pre=self.poisson, post=self.lif0, conn=nn.One2One(), weight=1000.)
self.dc_conn = nn.StaticSynapse(self.dc, self.lif1, conn=nn.One2One())
self.synapse1 = nn.StaticSynapse(
pre=self.lif0,
post=self.lif1,
conn=nn.FixedTotalNum(80, multi_conn=False),
conn_repr=ConnectRepr.MAT,
weight=5.,
delay_step=2)
class SNetwork(nn.Module):
def setup(self):
self.network = nn.SNetLayer(SimNet)
def __call__(self, input=None, t=0, monitor=None):
_, mon = self.network(input=input, t=t, monitor=monitor)
return mon
net = SNetwork()
以上网络中,在 setup 函数中写入了神经元和突触的定义。
NeurAI
框架支持多种神经元模型,具体可参考 神经元模型, 也支持多种突触模型,具体可参考 突触模型。
SNet 和 SNetLayer 介绍#
为保持接口统一性和简洁,使用 neurai.nn.snet.SNet
来构建网络,此类继承自 neurai.nn.module.Module
, neurai.nn.snet.SNet
是一个神经网络的抽象类,它包含了神经元和突触的更新逻辑。 neurai.nn.snet.SNetLayer
则是 SNet 的一个封装,它将 SNet 的网络作为参数,并提供网络的输入输出接口, 在其中包含了更多的处理细节,比如即时编译分区扫描,监视器等。处理了即时编译分区扫描以及监视器相关的逻辑。
使用监视器#
可以创建监视器来记录和观察在仿真过程中的电压和脉冲,如果有 STDP 连接,还可以记录 STDP 权重的变化。
from neurai.monitor import MonitorBS, MonitorConf, MemoryRecorder
monitorbs = MonitorBS(monitors=[MonitorConf("ExpLIF_0", "spike"), MonitorConf("ExpLIF_0", "v")], recorder=MemoryRecorder())
有关监视器的详细信息,可参考 监视神经元及突触信息。
运行网络#
使用 init 函数初始化模型,然后使用 run 函数运行仿真,并传入监视器。
sim_t = 5.0
dt = 0.1
param = net.init()
mon = net.run(param, t=sim_t, monitor=monitorbs)
数据可视化#
可以使用 neurai.util.visualization
模块来可视化网络的输出结果:
from neurai.util import visualization
import jax.numpy as jnp
visualization.raster_plot(
mon["ts"], jnp.asarray(mon['ExpLIF_0.spike']), show=True, save=True, title="ExpLIF_0.spike")
visualization.line_plot(
mon["ts"], jnp.asarray(mon['ExpLIF_0.v'])[:, 0], xlabel="Time(ms)", ylabel="ExpLIF_0.V(mv)", show=True, save=True, title="ExpLIF_0.v")
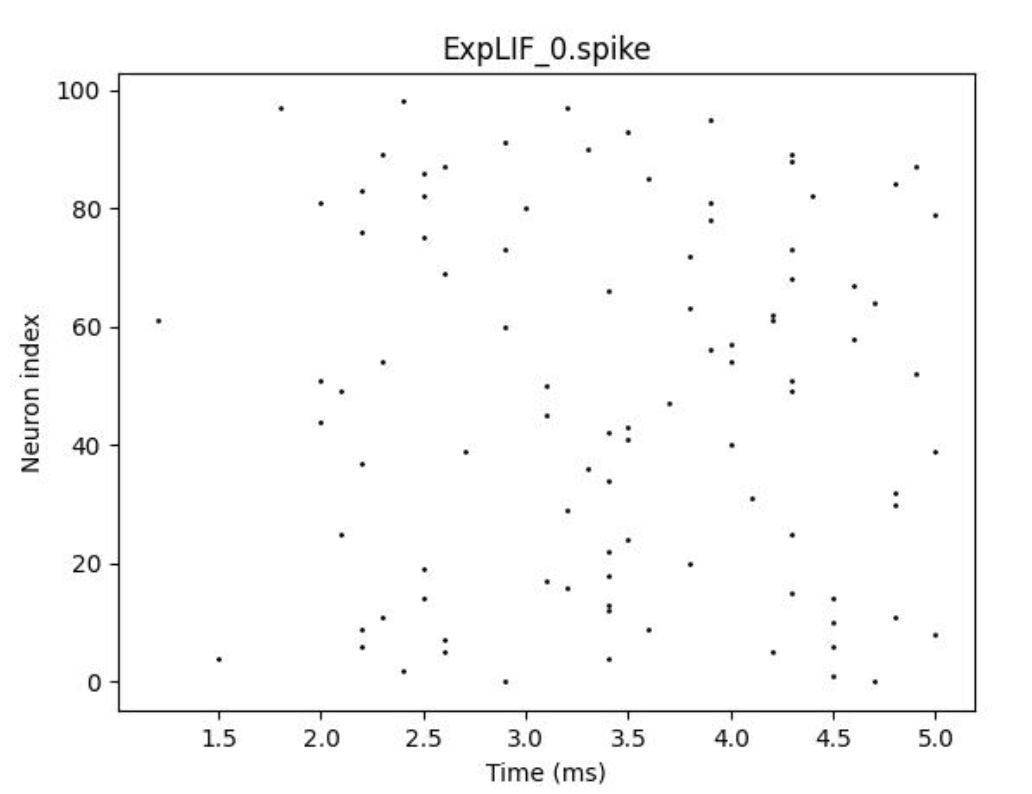
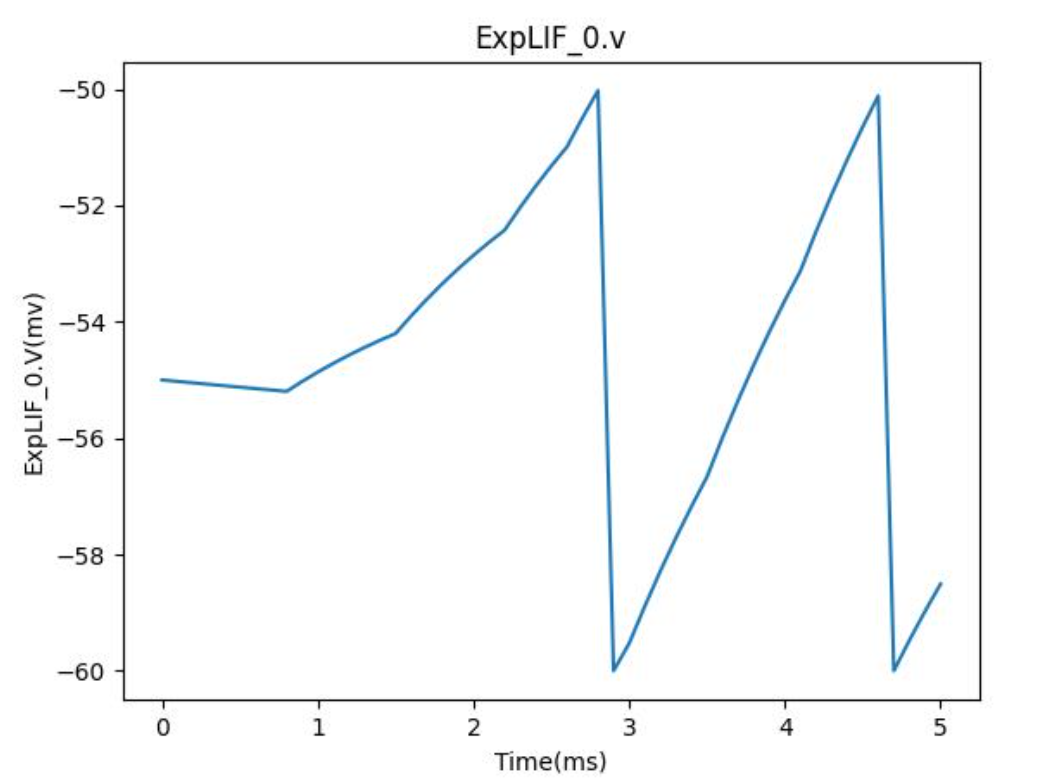
完整代码#
from neurai.config import set_platform
from neurai import nn
from neurai.const import ConnectRepr
from neurai.util import visualization
import jax.numpy as jnp
from neurai.monitor import MonitorBS, MonitorConf, MemoryRecorder
set_platform('gpu')
class SimNet(nn.SNet):
def setup(self):
self.lif0 = nn.ExpLIF(size=100, v_init=-55, V_rest=-60., V_th=-50., V_reset=-60., tau=20., I_e=0.)
self.lif1 = nn.ExpLIF(size=100, v_init=-55, V_rest=-60., V_th=-50., V_reset=-60., tau=20., I_e=0.)
self.poisson = nn.PoissonGenerator(size=100, rate=1000)
self.dc = nn.DCGenerator(size=100, amplitude=50, start=0.5, stop=1.5)
self.synapse0 = nn.StaticSynapse(pre=self.poisson, post=self.lif0, conn=nn.One2One(), weight=1000.)
self.dc_conn = nn.StaticSynapse(self.dc, self.lif1, conn=nn.One2One())
self.synapse1 = nn.StaticSynapse(
pre=self.lif0,
post=self.lif1,
conn=nn.FixedTotalNum(80, multi_conn=False),
conn_repr=ConnectRepr.MAT,
weight=5.,
delay_step=2)
class SNetwork(nn.Module):
def setup(self):
self.network = nn.SNetLayer(SimNet)
def __call__(self, input=None, t=0, monitor=None):
_, mon = self.network(input=input, t=t, monitor=monitor)
return mon
net = SNetwork()
sim_t = 5.0
dt = 0.1
monitorbs = MonitorBS(monitors=[MonitorConf("ExpLIF_0", "spike"), MonitorConf("ExpLIF_0", "v")], recorder=MemoryRecorder())
param = net.init()
mon = net.run(param, t=sim_t, monitor=monitorbs)
visualization.raster_plot(mon["ts"], jnp.asarray(mon['ExpLIF_0.spike']), show=True, save=True, title="ExpLIF_0.spike")
visualization.line_plot(
mon["ts"],
jnp.asarray(mon['ExpLIF_0.v'])[:, 0],
xlabel="Time(ms)",
ylabel="ExpLIF_0.V(mv)",
show=True,
save=True,
title="ExpLIF_0.v")